Deeplearning.ai’s “Advanced Retrieval for AI with Chroma” course’s Turkish translation.
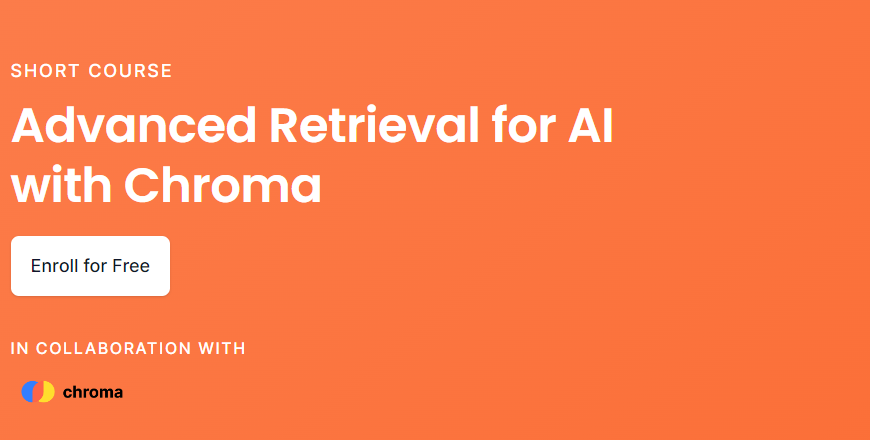
Simple RAG architecture is useful, but it can be improved. Query expansion rewrites the query to include more directly relevant documents. Two essential related strategies exist: one is to expand the optional question into several queries by rewording or rewriting it in various ways. Second, we can imagine or hypothesize what the answer would look like to see if we can uncover anything in our document collection that resembles an answer rather than simply discussing the query's themes.
This movie is sponsored by Chroma, which supplies the most popular vector datasets. This post will teach you some strategies for improving the results of your RAG apps. The first way employs an LLM to enhance the question itself. Another method reranks query results using a cross encoder, which takes two sentences and generates a relevancy score. You will also learn how to modify the query embeddings based on user feedback in order to generate a more appropriate score. Finally, we will consider emerging approaches that are not yet widespread.
Overview of Embeddings-Based Retrieval
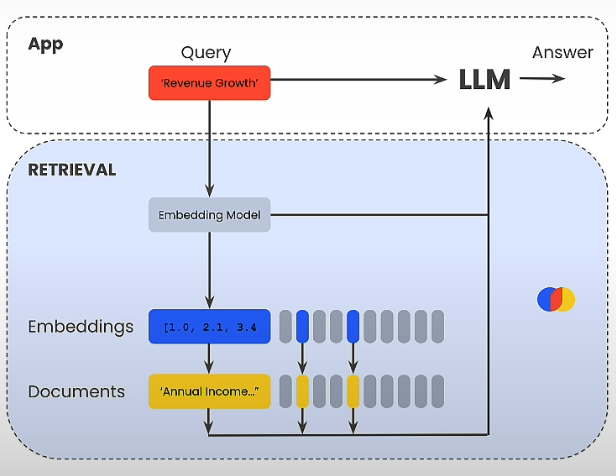
Encoder language models, like as BERT, can translate words into float values known as embeddings. Sentence embedding models, such as Sentence-BERT, employ Siamese. BERT-Networks may combine the embeddings of individual words in a sentence and assign embedding values to sentences.
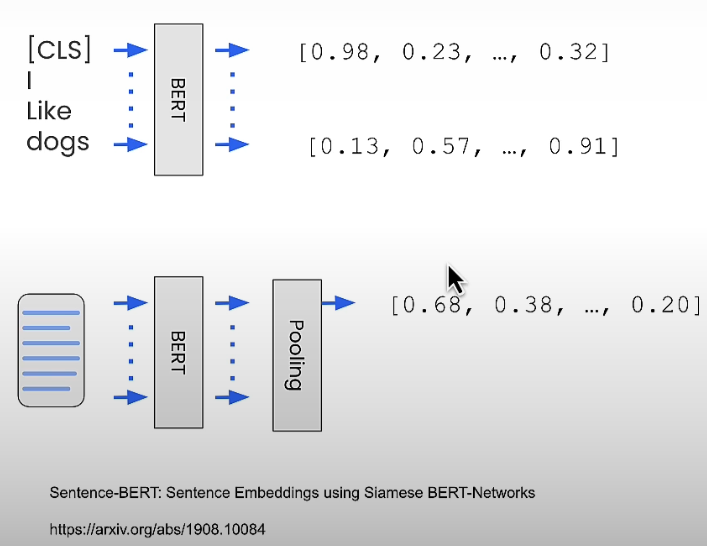
Below is the code of a conventional RAG architecture:
from helper_utils import word_wrap
from pypdf import PdfReader
reader = PdfReader("microsoft_annual_report_2022.pdf")
pdf_texts = [p.extract_text().strip() for p in reader.pages]
# Filter the empty strings
pdf_texts = [text for text in pdf_texts if text]
print(word_wrap(pdf_texts[0]))
from langchain.text_splitter import RecursiveCharacterTextSplitter, SentenceTransformersTokenTextSplitter
character_splitter = RecursiveCharacterTextSplitter(
separators=["\n\n", "\n", ". ", " ", ""],
chunk_size=1000,
chunk_overlap=0
)
character_split_texts = character_splitter.split_text('\n\n'.join(pdf_texts))
print(word_wrap(character_split_texts[10]))
print(f"\nTotal chunks: {len(character_split_texts)}")
token_splitter = SentenceTransformersTokenTextSplitter(chunk_overlap=0, tokens_per_chunk=256)
token_split_texts = []
for text in character_split_texts:
token_split_texts += token_splitter.split_text(text)
print(word_wrap(token_split_texts[10]))
print(f"\nTotal chunks: {len(token_split_texts)}")
import chromadb
from chromadb.utils.embedding_functions import SentenceTransformerEmbeddingFunction
embedding_function = SentenceTransformerEmbeddingFunction()
print(embedding_function([token_split_texts[10]]))
chroma_client = chromadb.Client()
chroma_collection = chroma_client.create_collection("microsoft_annual_report_2022", embedding_function=embedding_function)
ids = [str(i) for i in range(len(token_split_texts))]
chroma_collection.add(ids=ids, documents=token_split_texts)
chroma_collection.count()
query = "What was the total revenue?"
results = chroma_collection.query(query_texts=[query], n_results=5)
retrieved_documents = results['documents'][0]
for document in retrieved_documents:
print(word_wrap(document))
print('\n')
import os
import openai
from openai import OpenAI
from dotenv import load_dotenv, find_dotenv
_ = load_dotenv(find_dotenv()) # read local .env file
openai.api_key = os.environ['OPENAI_API_KEY']
openai_client = OpenAI()
def rag(query, retrieved_documents, model="gpt-3.5-turbo"):
information = "\n\n".join(retrieved_documents)
messages = [
{
"role": "system",
"content": "You are a helpful expert financial research assistant. Your users are asking questions about information contained in an annual report."
"You will be shown the user's question, and the relevant information from the annual report. Answer the user's question using only this information."
},
{"role": "user", "content": f"Question: {query}. \n Information: {information}"}
]
response = openai_client.chat.completions.create(
model=model,
messages=messages,
)
content = response.choices[0].message.content
return content
output = rag(query=query, retrieved_documents=retrieved_documents)
print(word_wrap(output))
Pitfalls of Retrieval When Simple Vector Searches Fails
We'll look at few scenarios when simple vector searches fail. Embeddings and vectors are geometric data structures that can be reasoned about spatially. Embeddings are incredibly high dimensional, with 348 dimensions, yet we can project them down to two dimensions that humans can see. To accomplish this, we will use UMAP. UMAP, which stands for Uniform Manifold Approximation, is an open-source library that may be used to project high-dimensional data into two or three dimensions for visualization. UMAP is a comparable technique to PCA or t-SNE, except UMAP specifically seeks to retain the structure of the data in terms of distances between points as much as it can, whereas PCA tries to determine the dominating directions and project data down in that way.
from helper_utils import load_chroma, word_wrap
from chromadb.utils.embedding_functions import SentenceTransformerEmbeddingFunction
embedding_function = SentenceTransformerEmbeddingFunction()
chroma_collection = load_chroma(filename='microsoft_annual_report_2022.pdf', collection_name='microsoft_annual_report_2022', embedding_function=embedding_function)
chroma_collection.count()
import umap
import numpy as np
from tqdm import tqdm
embeddings = chroma_collection.get(include=['embeddings'])['embeddings']
umap_transform = umap.UMAP(random_state=0, transform_seed=0).fit(embeddings)
def project_embeddings(embeddings, umap_transform):
umap_embeddings = np.empty((len(embeddings),2))
for i, embedding in enumerate(tqdm(embeddings)):
umap_embeddings[i] = umap_transform.transform([embedding])
return umap_embeddings
projected_dataset_embeddings = project_embeddings(embeddings, umap_transform)
import matplotlib.pyplot as plt
plt.figure()
plt.scatter(projected_dataset_embeddings[:, 0], projected_dataset_embeddings[:, 1], s=10)
plt.gca().set_aspect('equal', 'datalim')
plt.title('Projected Embeddings')
plt.axis('off')

Relevancy and Distraction
query = "What is the total revenue?"
results = chroma_collection.query(query_texts=query, n_results=5, include=['documents', 'embeddings'])
retrieved_documents = results['documents'][0]
for document in results['documents'][0]:
print(word_wrap(document))
print('')
query_embedding = embedding_function([query])[0]
retrieved_embeddings = results['embeddings'][0]
projected_query_embedding = project_embeddings([query_embedding], umap_transform)
projected_retrieved_embeddings = project_embeddings(retrieved_embeddings, umap_transform)
# Plot the projected query and retrieved documents in the embedding space
plt.figure()
plt.scatter(projected_dataset_embeddings[:, 0], projected_dataset_embeddings[:, 1], s=10, color='gray')
plt.scatter(projected_query_embedding[:, 0], projected_query_embedding[:, 1], s=150, marker='X', color='r')
plt.scatter(projected_retrieved_embeddings[:, 0], projected_retrieved_embeddings[:, 1], s=100, facecolors='none', edgecolors='g')
plt.gca().set_aspect('equal', 'datalim')
plt.title(f'{query}')
plt.axis('off')
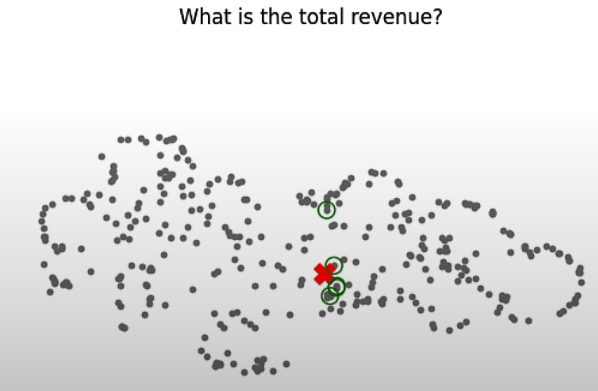
query = "What is the strategy around artificial intelligence (AI) ?"
results = chroma_collection.query(query_texts=query, n_results=5, include=['documents', 'embeddings'])
retrieved_documents = results['documents'][0]
for document in results['documents'][0]:
print(word_wrap(document))
print('')
query_embedding = embedding_function([query])[0]
retrieved_embeddings = results['embeddings'][0]
projected_query_embedding = project_embeddings([query_embedding], umap_transform)
projected_retrieved_embeddings = project_embeddings(retrieved_embeddings, umap_transform)
# Plot the projected query and retrieved documents in the embedding space
plt.figure()
plt.scatter(projected_dataset_embeddings[:, 0], projected_dataset_embeddings[:, 1], s=10, color='gray')
plt.scatter(projected_query_embedding[:, 0], projected_query_embedding[:, 1], s=150, marker='X', color='r')
plt.scatter(projected_retrieved_embeddings[:, 0], projected_retrieved_embeddings[:, 1], s=100, facecolors='none', edgecolors='g')
plt.gca().set_aspect('equal', 'datalim')
plt.title(f'{query}')
plt.axis('off')
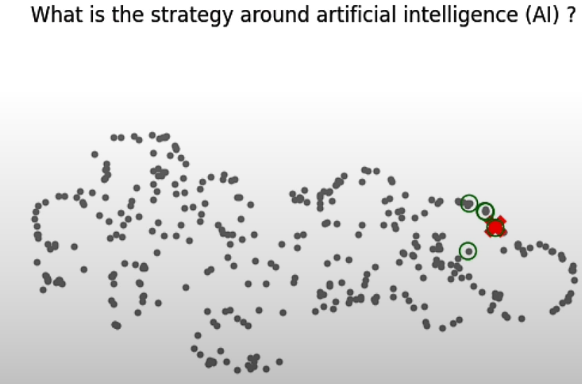
query = "What has been the investment in research and development?"
results = chroma_collection.query(query_texts=query, n_results=5, include=['documents', 'embeddings'])
retrieved_documents = results['documents'][0]
for document in results['documents'][0]:
print(word_wrap(document))
print('')
query_embedding = embedding_function([query])[0]
retrieved_embeddings = results['embeddings'][0]
projected_query_embedding = project_embeddings([query_embedding], umap_transform)
projected_retrieved_embeddings = project_embeddings(retrieved_embeddings, umap_transform)
# Plot the projected query and retrieved documents in the embedding space
plt.figure()
plt.scatter(projected_dataset_embeddings[:, 0], projected_dataset_embeddings[:, 1], s=10, color='gray')
plt.scatter(projected_query_embedding[:, 0], projected_query_embedding[:, 1], s=150, marker='X', color='r')
plt.scatter(projected_retrieved_embeddings[:, 0], projected_retrieved_embeddings[:, 1], s=100, facecolors='none', edgecolors='g')
plt.gca().set_aspect('equal', 'datalim')
plt.title(f'{query}')
plt.axis('off')
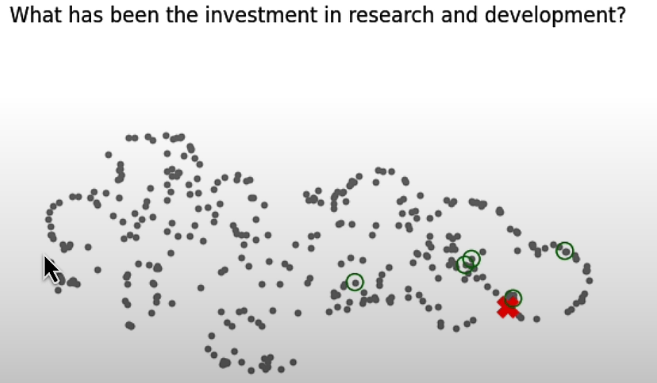
query = "What has Michael Jordan done for us lately?"
results = chroma_collection.query(query_texts=query, n_results=5, include=['documents', 'embeddings'])
retrieved_documents = results['documents'][0]
for document in results['documents'][0]:
print(word_wrap(document))
print('')
query_embedding = embedding_function([query])[0]
retrieved_embeddings = results['embeddings'][0]
projected_query_embedding = project_embeddings([query_embedding], umap_transform)
projected_retrieved_embeddings = project_embeddings(retrieved_embeddings, umap_transform)
# Plot the projected query and retrieved documents in the embedding space
plt.figure()
plt.scatter(projected_dataset_embeddings[:, 0], projected_dataset_embeddings[:, 1], s=10, color='gray')
plt.scatter(projected_query_embedding[:, 0], projected_query_embedding[:, 1], s=150, marker='X', color='r')
plt.scatter(projected_retrieved_embeddings[:, 0], projected_retrieved_embeddings[:, 1], s=100, facecolors='none', edgecolors='g')
plt.gca().set_aspect('equal', 'datalim')
plt.title(f'{query}')
plt.axis('off')
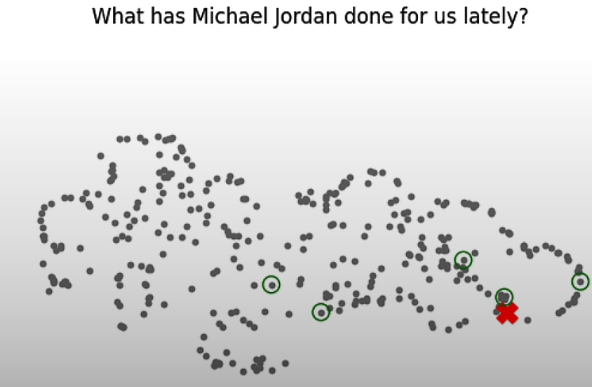
Query Expansion
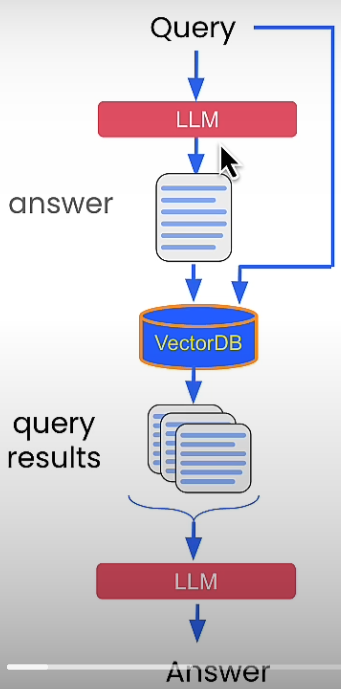
from helper_utils import load_chroma, word_wrap, project_embeddings
from chromadb.utils.embedding_functions import SentenceTransformerEmbeddingFunction
embedding_function = SentenceTransformerEmbeddingFunction()
chroma_collection = load_chroma(filename='microsoft_annual_report_2022.pdf', collection_name='microsoft_annual_report_2022', embedding_function=embedding_function)
chroma_collection.count()
import os
import openai
from openai import OpenAI
from dotenv import load_dotenv, find_dotenv
_ = load_dotenv(find_dotenv()) # read local .env file
openai.api_key = os.environ['OPENAI_API_KEY']
openai_client = OpenAI()
import umap
embeddings = chroma_collection.get(include=['embeddings'])['embeddings']
umap_transform = umap.UMAP(random_state=0, transform_seed=0).fit(embeddings)
projected_dataset_embeddings = project_embeddings(embeddings, umap_transform)
Expansion with generated answers
Query expansion is a widely used technique to improve the recall of search systems. In this paper, we propose an…arxiv.org
def augment_query_generated(query, model="gpt-3.5-turbo"):
messages = [
{
"role": "system",
"content": "You are a helpful expert financial research assistant. Provide an example answer to the given question, that might be found in a document like an annual report. "
},
{"role": "user", "content": query}
]
response = openai_client.chat.completions.create(
model=model,
messages=messages,
)
content = response.choices[0].message.content
return content
original_query = "Was there significant turnover in the executive team?"
hypothetical_answer = augment_query_generated(original_query)
joint_query = f"{original_query} {hypothetical_answer}"
print(word_wrap(joint_query))
results = chroma_collection.query(query_texts=joint_query, n_results=5, include=['documents', 'embeddings'])
retrieved_documents = results['documents'][0]
for doc in retrieved_documents:
print(word_wrap(doc))
print('')
retrieved_embeddings = results['embeddings'][0]
original_query_embedding = embedding_function([original_query])
augmented_query_embedding = embedding_function([joint_query])
projected_original_query_embedding = project_embeddings(original_query_embedding, umap_transform)
projected_augmented_query_embedding = project_embeddings(augmented_query_embedding, umap_transform)
projected_retrieved_embeddings = project_embeddings(retrieved_embeddings, umap_transform)
import matplotlib.pyplot as plt
# Plot the projected query and retrieved documents in the embedding space
plt.figure()
plt.scatter(projected_dataset_embeddings[:, 0], projected_dataset_embeddings[:, 1], s=10, color='gray')
plt.scatter(projected_retrieved_embeddings[:, 0], projected_retrieved_embeddings[:, 1], s=100, facecolors='none', edgecolors='g')
plt.scatter(projected_original_query_embedding[:, 0], projected_original_query_embedding[:, 1], s=150, marker='X', color='r')
plt.scatter(projected_augmented_query_embedding[:, 0], projected_augmented_query_embedding[:, 1], s=150, marker='X', color='orange')
plt.gca().set_aspect('equal', 'datalim')
plt.title(f'{original_query}')
plt.axis('off')
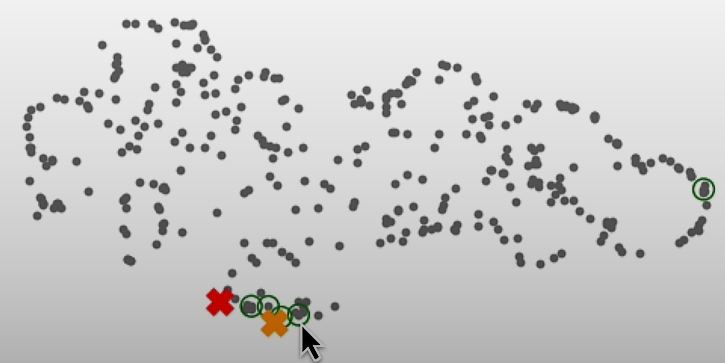
Expansion with Multiple Queries
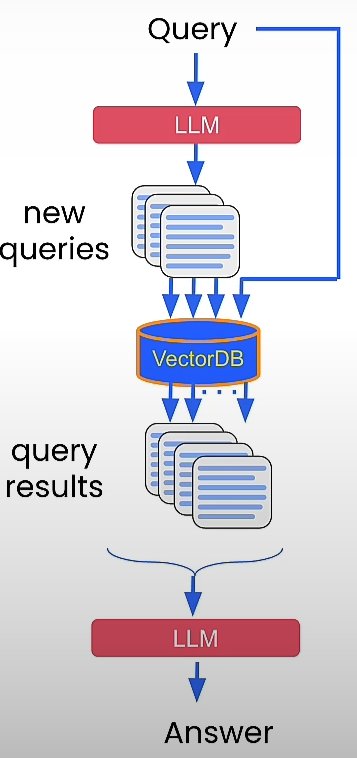
def augment_multiple_query(query, model="gpt-3.5-turbo"):
messages = [
{
"role": "system",
"content": "You are a helpful expert financial research assistant. Your users are asking questions about an annual report. "
"Suggest up to five additional related questions to help them find the information they need, for the provided question. "
"Suggest only short questions without compound sentences. Suggest a variety of questions that cover different aspects of the topic."
"Make sure they are complete questions, and that they are related to the original question."
"Output one question per line. Do not number the questions."
},
{"role": "user", "content": query}
]
response = openai_client.chat.completions.create(
model=model,
messages=messages,
)
content = response.choices[0].message.content
content = content.split("\n")
return content
original_query = "What were the most important factors that contributed to increases in revenue?"
augmented_queries = augment_multiple_query(original_query)
for query in augmented_queries:
print(query)
queries = [original_query] + augmented_queries
results = chroma_collection.query(query_texts=queries, n_results=5, include=['documents', 'embeddings'])
retrieved_documents = results['documents']
# Deduplicate the retrieved documents
unique_documents = set()
for documents in retrieved_documents:
for document in documents:
unique_documents.add(document)
for i, documents in enumerate(retrieved_documents):
print(f"Query: {queries[i]}")
print('')
print("Results:")
for doc in documents:
print(word_wrap(doc))
print('')
print('-'*100)
original_query_embedding = embedding_function([original_query])
augmented_query_embeddings = embedding_function(augmented_queries)
project_original_query = project_embeddings(original_query_embedding, umap_transform)
project_augmented_queries = project_embeddings(augmented_query_embeddings, umap_transform)
result_embeddings = results['embeddings']
result_embeddings = [item for sublist in result_embeddings for item in sublist]
projected_result_embeddings = project_embeddings(result_embeddings, umap_transform)
import matplotlib.pyplot as plt
plt.figure()
plt.scatter(projected_dataset_embeddings[:, 0], projected_dataset_embeddings[:, 1], s=10, color='gray')
plt.scatter(project_augmented_queries[:, 0], project_augmented_queries[:, 1], s=150, marker='X', color='orange')
plt.scatter(projected_result_embeddings[:, 0], projected_result_embeddings[:, 1], s=100, facecolors='none', edgecolors='g')
plt.scatter(project_original_query[:, 0], project_original_query[:, 1], s=150, marker='X', color='r')
plt.gca().set_aspect('equal', 'datalim')
plt.title(f'{original_query}')
plt.axis('off')
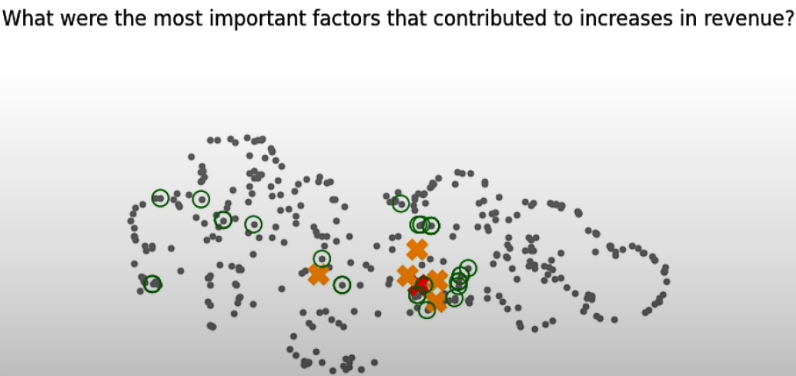
By doing so, we were able to cover additional aspects of our datasets, including the answer to our inquiry.
Crossencoder Reranking
The Crossencoder reranks the relevance of our received results for the query we supplied. Reranking reorders them based on their relevance to a certain query.
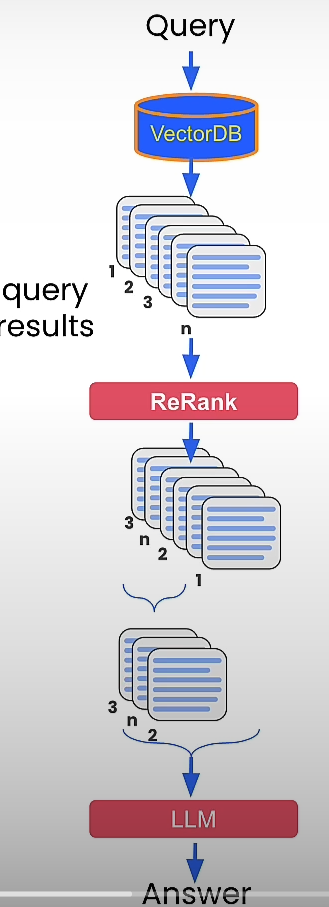
from helper_utils import load_chroma, word_wrap, project_embeddings
from chromadb.utils.embedding_functions import SentenceTransformerEmbeddingFunction
import numpy as np
embedding_function = SentenceTransformerEmbeddingFunction()
chroma_collection = load_chroma(filename='microsoft_annual_report_2022.pdf', collection_name='microsoft_annual_report_2022', embedding_function=embedding_function)
chroma_collection.count()
Re-ranking the long tail
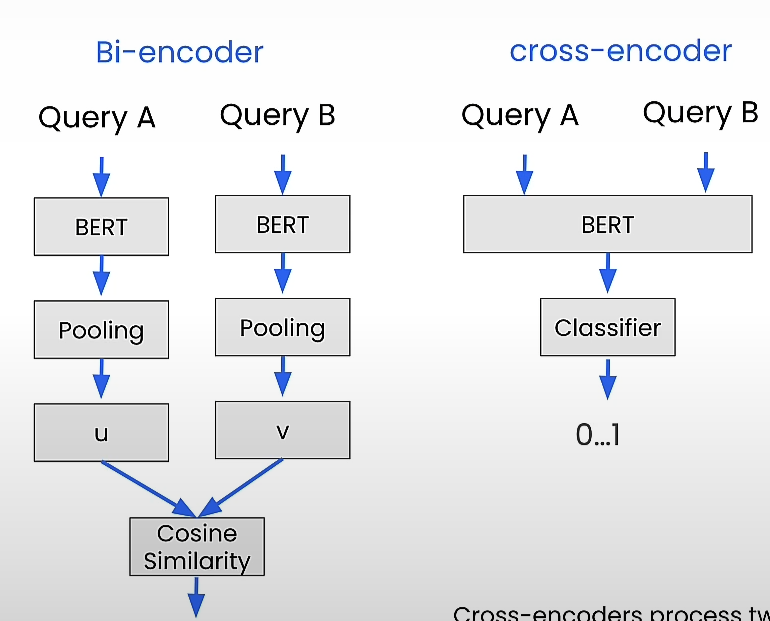
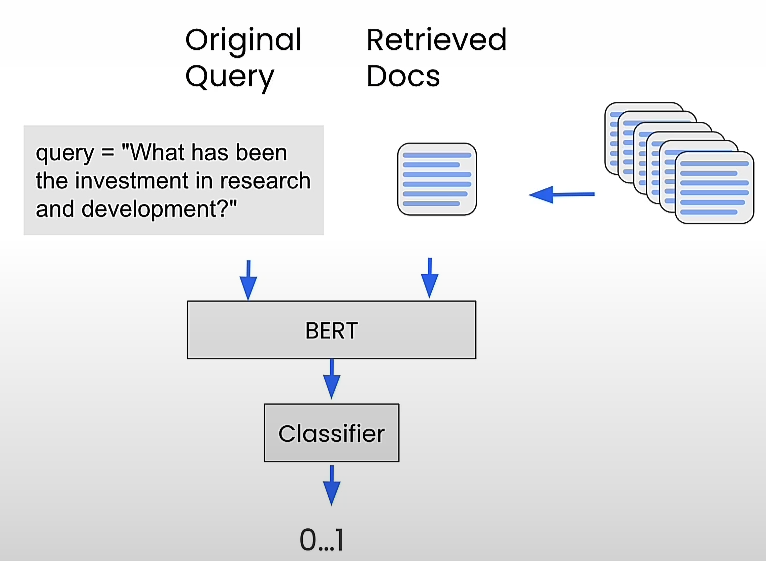
query = "What has been the investment in research and development?"
results = chroma_collection.query(query_texts=query, n_results=10, include=['documents', 'embeddings'])
retrieved_documents = results['documents'][0]
for document in results['documents'][0]:
print(word_wrap(document))
print('')
from sentence_transformers import CrossEncoder
cross_encoder = CrossEncoder('cross-encoder/ms-marco-MiniLM-L-6-v2')
pairs = [[query, doc] for doc in retrieved_documents]
scores = cross_encoder.predict(pairs)
print("Scores:")
for score in scores:
print(score) # First 2 docs have high score, all the second retrieved doc is much higher than the first one
print("New Ordering:")
for o in np.argsort(scores)[::-1]:
print(o+1)
Re-ranking with Query Expansion
Instead of simply providing all of the enhanced extended queries to the LLM, we can use cross-reranking to extract all of the best results for the original query.
original_query = "What were the most important factors that contributed to increases in revenue?"
generated_queries = [
"What were the major drivers of revenue growth?",
"Were there any new product launches that contributed to the increase in revenue?",
"Did any changes in pricing or promotions impact the revenue growth?",
"What were the key market trends that facilitated the increase in revenue?",
"Did any acquisitions or partnerships contribute to the revenue growth?"
]
queries = [original_query] + generated_queries
results = chroma_collection.query(query_texts=queries, n_results=10, include=['documents', 'embeddings'])
retrieved_documents = results['documents']
# Deduplicate the retrieved documents
unique_documents = set()
for documents in retrieved_documents:
for document in documents:
unique_documents.add(document)
unique_documents = list(unique_documents)
pairs = []
for doc in unique_documents:
pairs.append([original_query, doc])
scores = cross_encoder.predict(pairs)
print("Scores:")
for score in scores:
print(score)
print("New Ordering:")
for o in np.argsort(scores)[::-1]:
print(o)
We learnt how to utilize a cross-encoder as a re-ranking model, and we saw how to use re-ranking to get more out of a single query's long tail, as well as to filter the results of an augmented enlarged query to only the results relevant to the original query.
Embedding Adaptors
Let's take a look at how we can leverage user feedback on the relevance of retrieved results to automatically improve the retrieval system's performance using a technique known as embedded adapters. Embedding adapters is a method of directly embedding a query to improve retrieval performance. In effect, we introduce an additional stage in the retrieval system known as an embedding adapter, which occurs after the embedding model but before we get the most relevant results. We train the embedding adapter based on user feedback on the relevance of our obtained results for a set of searches.
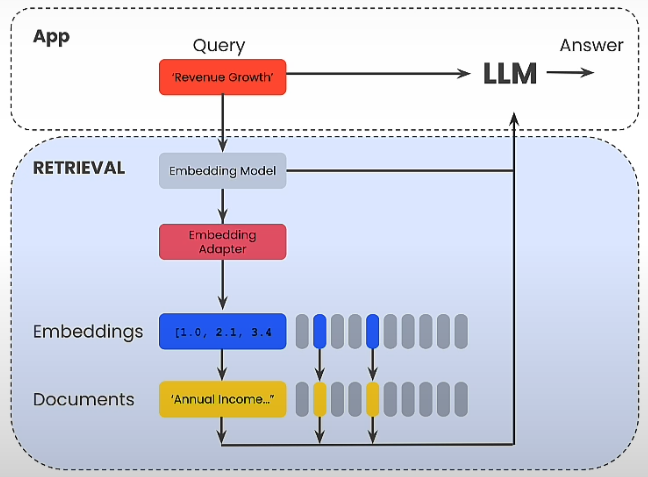
from helper_utils import load_chroma, word_wrap, project_embeddings
from chromadb.utils.embedding_functions import SentenceTransformerEmbeddingFunction
import numpy as np
import umap
from tqdm import tqdm
import torch
embedding_function = SentenceTransformerEmbeddingFunction()
chroma_collection = load_chroma(filename='microsoft_annual_report_2022.pdf', collection_name='microsoft_annual_report_2022', embedding_function=embedding_function)
chroma_collection.count()
embeddings = chroma_collection.get(include=['embeddings'])['embeddings']
umap_transform = umap.UMAP(random_state=0, transform_seed=0).fit(embeddings)
projected_dataset_embeddings = project_embeddings(embeddings, umap_transform)
import os
import openai
from openai import OpenAI
from dotenv import load_dotenv, find_dotenv
_ = load_dotenv(find_dotenv()) # read local .env file
openai.api_key = os.environ['OPENAI_API_KEY']
openai_client = OpenAI()
Creating a dataset
This technique requires a dataset. We did not have any people using our RAG application, thus we have no data. We can have a model generate a data set for us. The key here is to come up with the appropriate prompt. We are effectively prompting the model to act as an expert, helpful financial research assistant. This should include 10 to 15 short questions to ask when assessing an annual report, as well as recommendations for the output. This will produce several queries that users may have already run against our system.
def generate_queries(model="gpt-3.5-turbo"):
messages = [
{
"role": "system",
"content": "You are a helpful expert financial research assistant. You help users analyze financial statements to better understand companies. "
"Suggest 10 to 15 short questions that are important to ask when analyzing an annual report. "
"Do not output any compound questions (questions with multiple sentences or conjunctions)."
"Output each question on a separate line divided by a newline."
},
]
response = openai_client.chat.completions.create(
model=model,
messages=messages,
)
content = response.choices[0].message.content
content = content.split("\n")
return content
generated_queries = generate_queries()
for query in generated_queries:
print(query)
results = chroma_collection.query(query_texts=generated_queries, n_results=10, include=['documents', 'embeddings'])
retrieved_documents = results['documents']
def evaluate_results(query, statement, model="gpt-3.5-turbo"):
messages = [
{
"role": "system",
"content": "You are a helpful expert financial research assistant. You help users analyze financial statements to better understand companies. "
"For the given query, evaluate whether the following satement is relevant."
"Output only 'yes' or 'no'."
},
{
"role": "user",
"content": f"Query: {query}, Statement: {statement}"
}
]
response = openai_client.chat.completions.create(
model=model,
messages=messages,
max_tokens=1
)
content = response.choices[0].message.content
if content == "yes":
return 1
return -1
retrieved_embeddings = results['embeddings']
query_embeddings = embedding_function(generated_queries)
adapter_query_embeddings = []
adapter_doc_embeddings = []
adapter_labels = []
for q, query in enumerate(tqdm(generated_queries)):
for d, document in enumerate(retrieved_documents[q]):
adapter_query_embeddings.append(query_embeddings[q])
adapter_doc_embeddings.append(retrieved_embeddings[q][d])
adapter_labels.append(evaluate_results(query, document))
len(adapter_labels)
adapter_query_embeddings = torch.Tensor(np.array(adapter_query_embeddings))
adapter_doc_embeddings = torch.Tensor(np.array(adapter_doc_embeddings))
adapter_labels = torch.Tensor(np.expand_dims(np.array(adapter_labels),1))
dataset = torch.utils.data.TensorDataset(adapter_query_embeddings, adapter_doc_embeddings, adapter_labels)
Setting up the model
def model(query_embedding, document_embedding, adaptor_matrix):
updated_query_embedding = torch.matmul(adaptor_matrix, query_embedding)
return torch.cosine_similarity(updated_query_embedding, document_embedding, dim=0)
def mse_loss(query_embedding, document_embedding, adaptor_matrix, label):
return torch.nn.MSELoss()(model(query_embedding, document_embedding, adaptor_matrix), label)
# Initialize the adaptor matrix
mat_size = len(adapter_query_embeddings[0])
adapter_matrix = torch.randn(mat_size, mat_size, requires_grad=True)
min_loss = float('inf')
best_matrix = None
for epoch in tqdm(range(100)):
for query_embedding, document_embedding, label in dataset:
loss = mse_loss(query_embedding, document_embedding, adapter_matrix, label)
if loss < min_loss:
min_loss = loss
best_matrix = adapter_matrix.clone().detach().numpy()
loss.backward()
with torch.no_grad():
adapter_matrix -= 0.01 * adapter_matrix.grad
adapter_matrix.grad.zero_()
print(f"Best loss: {min_loss.detach().numpy()}")
test_vector = torch.ones((mat_size,1))
scaled_vector = np.matmul(best_matrix, test_vector).numpy()
import matplotlib.pyplot as plt
plt.bar(range(len(scaled_vector)), scaled_vector.flatten())
plt.show()
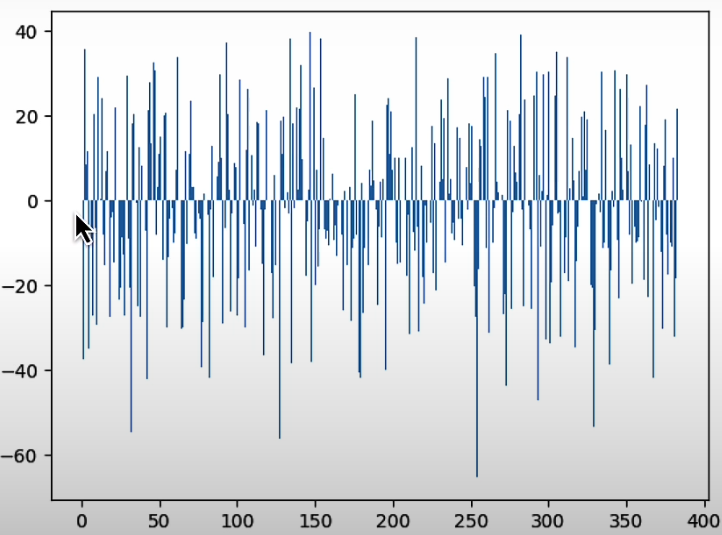
query_embeddings = embedding_function(generated_queries)
adapted_query_embeddings = np.matmul(best_matrix, np.array(query_embeddings).T).T
projected_query_embeddings = project_embeddings(query_embeddings, umap_transform)
projected_adapted_query_embeddings = project_embeddings(adapted_query_embeddings, umap_transform)
# Plot the projected query and retrieved documents in the embedding space
plt.figure()
plt.scatter(projected_dataset_embeddings[:, 0], projected_dataset_embeddings[:, 1], s=10, color='gray')
plt.scatter(projected_query_embeddings[:, 0], projected_query_embeddings[:, 1], s=150, marker='X', color='r', label="original")
plt.scatter(projected_adapted_query_embeddings[:, 0], projected_adapted_query_embeddings[:, 1], s=150, marker='X', color='green', label="adapted")
plt.gca().set_aspect('equal', 'datalim')
plt.title("Adapted Queries")
plt.axis('off')
plt.legend()
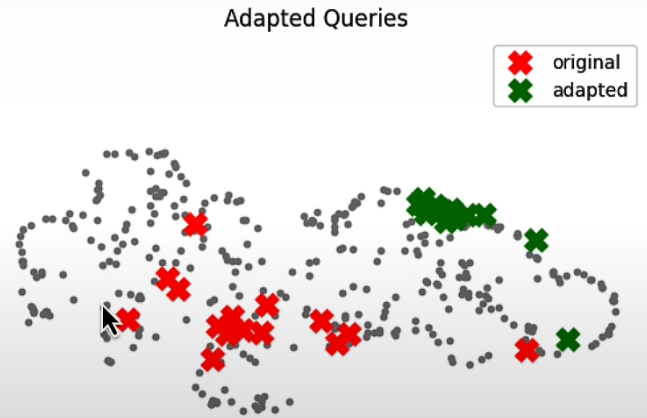
An embedding adaptor is a powerful and simple way to tailor query embeddings to your individual application. To make this work, you'll need to collect a dataset, either synthetic like the one we've created here or one based on user data. To make this work, you must collect a dataset, either synthetic, like the one we've created here, or one based on user data. User data works best because it indicates that people are utilizing your retrieval system for certain activities.
Other Techniques
RAG is still a fresh field where you can experiment with many ways. For example, you can fine-tune the embedding model directly with the same data that we used in the embedding lab. You can also adjust the llm for retrieval (see the RA-DIT and InstructRetro articles).
You can also experiment with a complex embedding adapter model that incorporates a full-blown neural network or even a transformer layer. Similarly, you can utilize a relevance modeling model instead of merely the cross-encoder. Finally, it is sometimes ignored that the quality of recovered results is determined by how your data is chunked before it is stored in the retrieval system.
Resource
[1] Deeplearning.ai, (2024), Advanced Retrieval for AI with Chroma:
[https://learn.deeplearning.ai/courses/advanced-retrieval-for-ai/lesson/1/introduction]
0 Comments